Precision Farming with GIS
Reading time
Content
Introduction to Precision Farming Concepts
Precision farming, also known as site-specific crop management or precision agriculture, represents a cutting-edge approach to modern agriculture. It integrates advanced technologies to optimise the use of resources, improve crop yields and minimise environmental impacts. Definitions of precision farming vary, but they converge on its core principles of efficiency and sustainability. Some define it as a management practice focused on utilising precise information to increase profitability. Others emphasize its environmental dimension, describing it as the application of technologies and principles to manage spatial and temporal variability for better crop performance and ecological benefits. From a technological perspective, it involves monitoring, analysing and controlling plant production to balance cost and ecological impact. Precision farming enhances resource efficiency by minimizing waste and reduces the environmental footprint of farming practices. An integrated view highlights the use of high-precision positioning systems, geospatial mapping and variable rate technologies.
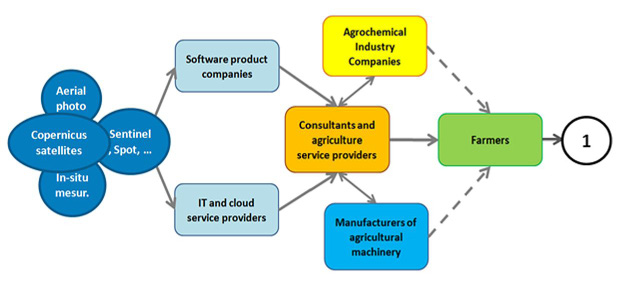
Actors in the field of precision farming. From data providers to farmers. Source: Šafář, V. et al. (2022). The Role of Remote Sensing in Agriculture and Future Vision. Agris On-line Papers in Economics and Informatics. XIV. 107-124. 10.7160/aol.2022.140109.
Precision farming relies on several key methods. Site-specific crop management tailors agricultural interventions to the unique characteristics of different zones within a field, using tools like GIS and remote sensing to map spatial variability. Variable rate technology enables the precise application of inputs such as fertilizers and pesticides, adjusted to the specific needs of various field areas. Remote sensing, through satellite and drone imagery, provides real-time insights into crop health, soil moisture and vegetation indices, enabling the early detection of stress factors such as nutrient deficiencies or diseases.
Another critical method is yield mapping, which combines GNSS with yield monitors on harvesting equipment to document spatial variations in productivity. This data informs future management strategies to enhance underperforming zones. Soil and crop sensing technologies measure vital parameters like soil moisture, nutrient content and pH levels, while advanced sensors assess crop nutrient levels and recommend suitable interventions.
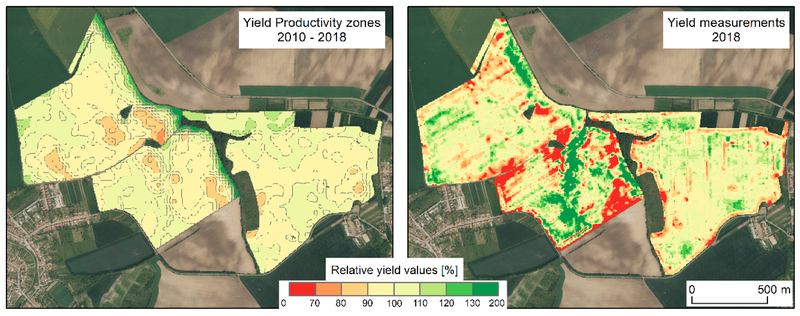
The analysis of yield productivity zones: yield productivity zone prediction derived from satellite images (left) and the map of yield measurements as computed by a harvester (right). Source: Řezník, T. et al. (2020). Prediction of Yield Productivity Zones from Landsat 8 and Sentinel-2A/B and Their Evaluation Using Farm Machinery Measurements. Remote Sensing, 12(12), 1917. https://doi.org/10.3390/rs12121917
Decision support systems play a central role in precision farming by integrating field data, weather forecasts and historical trends to optimize decisions on planting, irrigation and fertilisation. Geospatial analysis and mapping further enhance precision by providing detailed spatial layers, including soil types, elevation and water availability. The integration of Internet of Things (IoT) and big data analytics allows continuous data collection from the field, offering actionable insights that improve precision in resource allocation and intervention timing.
Methods of Precision Farming
Precision farming employs a variety of advanced methods designed to optimise agricultural practices, increase efficiency and minimize environmental impacts.
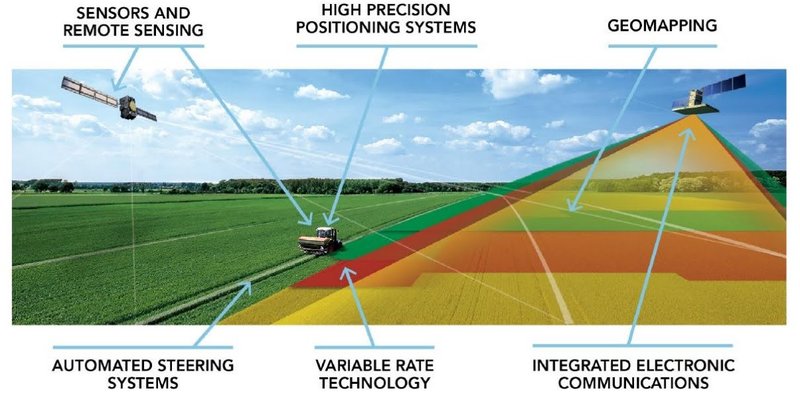
Technologies of precision farming. Source: https://kas32.com/en/post/view/332
One of the fundamental methods is site-specific crop management (SSCM), which involves tailoring agricultural practices to the unique conditions of specific zones within a field. This method is enabled by detailed mapping of spatial variability using geospatial technologies like GIS and remote sensing. By understanding differences in soil composition, moisture levels and nutrient availability, SSCM allows for customized interventions in each zone.
Variable Rate Technology (VRT) is another cornerstone of precision farming. It adjusts the application of inputs such as seeds, fertilisers and pesticides according to the precise requirements of different areas within a field. This technique reduces input waste, enhances crop productivity, and minimises the risk of overapplication, which can harm the environment.
Remote sensing plays a critical role in providing real-time data about crop health, soil moisture and vegetation status. Through satellite, aerial or drone imagery, remote sensing technologies enable farmers to monitor large areas quickly and detect potential problems such as nutrient deficiencies, pest infestations or water stress early. This timely information supports better decision-making and targeted interventions.
Yield mapping involves the use of GNSS-enabled yield monitors on harvesting equipment to record the spatial variations in crop output across a field. These maps help identify high- and low-performing areas, providing insights for optimizing future farming practices and addressing specific issues that limit productivity.
Soil and crop sensing technologies are crucial for understanding the precise conditions of the field. Sensors measure critical parameters such as soil pH, moisture levels, organic matter content and nutrient availability. Crop sensors assess the health and nutrient needs of plants, guiding variable-rate application of inputs.
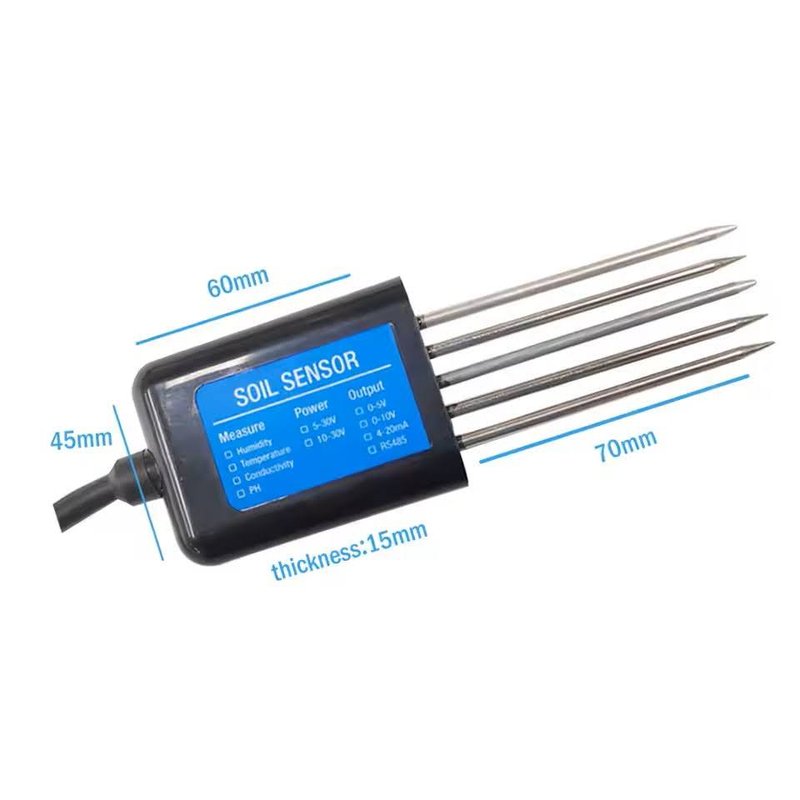
An integrated soil sensor measuring soil pH, moisture, temperature and conductivity.
Decision support systems (DSS) integrate data from various sources, such as weather forecasts, soil analysis and crop growth models, to provide actionable recommendations. These systems use advanced algorithms to help farmers make informed decisions about planting schedules, irrigation strategies and pest control measures.
Geospatial analysis and mapping enhance the precision of farming practices by generating detailed spatial layers of field attributes, such as elevation, soil type and water retention capacity. These layers inform planning and implementation, ensuring that resources are allocated optimally across the field.
The integration of Internet of Things (IoT) devices and big data analytics has revolutionised precision farming. IoT devices, such as soil moisture probes and climate stations, continuously collect field data. This data is processed using big data technologies to identify patterns and trends, improving the timing and accuracy of agricultural interventions.
Automation and robotics are increasingly utilized in precision farming, enabling tasks such as planting, spraying and harvesting to be performed with high accuracy. Automated machinery often operates using GNSS guidance, ensuring consistent performance and reducing human error.
Lastly, on-farm experimentation and adaptive management are integral to precision farming. Farmers use data to test and refine strategies, ensuring that practices remain responsive to changing conditions and field-specific dynamics.
Through these methods, precision farming achieves its goals of enhancing productivity, reducing costs and promoting environmental sustainability. By addressing the variability within fields and applying resources only where needed, these techniques ensure that modern agriculture meets the growing demands of a changing world.
Role of GIS in Precision Farming
Geographic Information Systems (GIS) are central to precision farming, enabling the efficient integration, analysis and visualization of spatial data to optimise agricultural practices. When combined with remote sensing, Internet of Things (IoT) data analysis, cloud computing and emerging GeoAI technologies, GIS transforms agricultural management into a data-driven, sustainable and future-ready domain.
GIS supports spatial mapping and analysis, creating detailed maps that reveal variability within fields, such as soil properties, moisture levels and crop health. These maps guide site-specific management, enabling precise interventions. Remote sensing enhances GIS by providing high-resolution imagery from satellites and Unmanned Aerial Vehicles (UAVs) to monitor vegetation health, detect diseases and assess water stress. For example, vegetation indices such as NDVI derived from remote sensing are processed in GIS to identify underperforming zones and direct resources where they are most needed.
The integration of IoT data analysis into GIS further expands its capabilities. IoT devices, including soil moisture sensors, weather stations and crop health monitors, generate vast quantities of real-time data. GIS serves as a platform to process and analyse this data spatially and temporally, transforming raw inputs into actionable insights. For example:
- Soil and Weather Monitoring: IoT data on soil moisture and weather conditions is overlaid in GIS to optimise irrigation schedules.
- Predictive Maintenance: Data from farm machinery is analysed to predict maintenance needs and prevent downtime.
- Dynamic Decision-Making: Real-time IoT data feeds into GIS-driven models to adjust field operations dynamically in response to changing conditions.
Data integration and cloud computing enhance the scalability and accessibility of GIS in precision farming. By storing and processing vast datasets on the cloud, GIS platforms support collaborative decision-making and enable stakeholders to access critical insights remotely. Cloud computing also powers advanced analytics, such as big data processing and predictive modeling.
GIS aids in decision-making and resource optimisation by combining spatial data with advanced predictive models. This ensures the precise application of inputs such as fertilisers and pesticides, reducing waste and costs. Variable rate technology, guided by GIS insights, applies resources only where needed, enhancing efficiency. Scenario analysis tools within GIS allow farmers to simulate various strategies, assessing their potential outcomes before implementation.
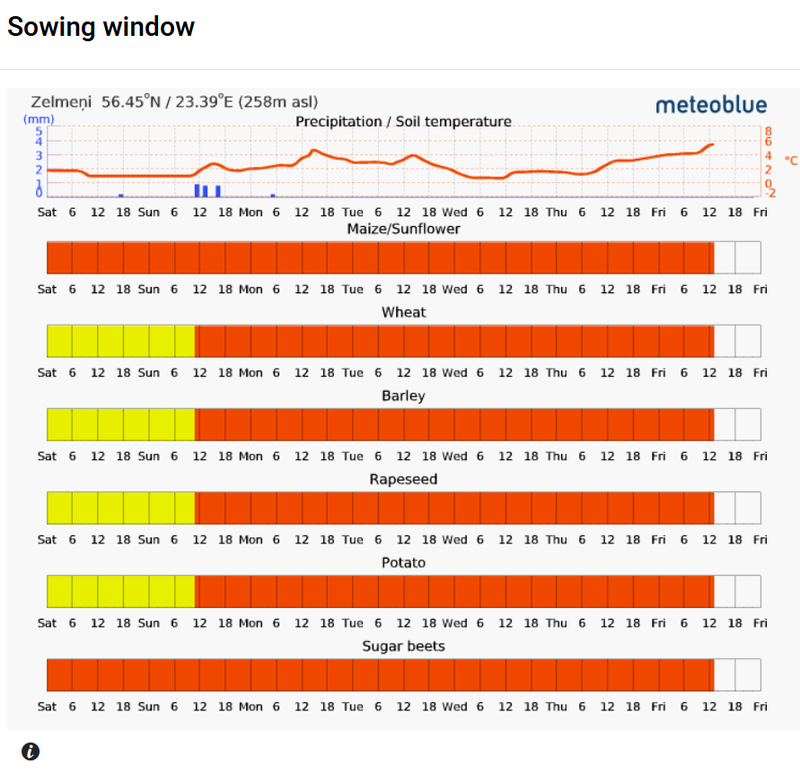
Based on a development of soil temperature and precipitation, the sowing window shows how suitable are time intervals in the upcoming 7 days for sowing different corn species in the area of interest. The scale goes from suitable (green) to unsuitable (red). Source: https://groundwater.smartagro.lv
Through continuous monitoring and feedback, GIS integrated with IoT and remote sensing data provides a dynamic view of field conditions. Real-time insights enable early detection of issues such as pest outbreaks or nutrient deficiencies. Additionally, GIS evaluates the effectiveness of applied practices, facilitating ongoing refinement and improvement.
GIS drives environmental and economic sustainability by reducing chemical usage, conserving water and maximising productivity. Targeted interventions minimize environmental impacts, while efficient resource use enhances profitability by lowering costs and improving yields.
Looking ahead, GeoAI (Geospatial Artificial Intelligence) is revolutionising GIS in precision farming. By leveraging machine learning and AI, GeoAI enables advanced analytics of geospatial and IoT data to identify patterns, predict trends and support real-time decision-making. For instance:
- Predictive Analytics: GeoAI forecasts crop yields, pest outbreaks and weather impacts with high precision.
- Automated Data Processing: AI algorithms process remote sensing and IoT data rapidly, providing actionable insights without manual intervention.
- Climate Resilience: GeoAI predicts the long-term impacts of climate change on agriculture, helping farmers adapt proactively.
The fusion of GIS, remote sensing, IoT data analysis, cloud computing and GeoAI is reshaping precision farming. These technologies enable modern agriculture to be more efficient, sustainable and resilient, addressing current challenges while preparing for future demands.
Exercise: Analysis of NDVI in QGIS
Problem to Solve: Create variable rate application zones for precision farming by analyzing crop health variability using the Normalized Difference Vegetation Index (NDVI) derived from remote sensing data. Identify areas of low, medium, and high vegetation health.
Data Needed:
- Remote Sensing Data – Multispectral imagery with at least two bands: red (R) and near-infrared (NIR). Data can come from satellites (e.g. Sentinel-2) or drones.
Tools in QGIS Processing Toolbox:
- Raster Calculator: To calculate NDVI.
- Reclassify by Table: To classify NDVI values into zones.
Workflow:
1. Load the Data
- Import the multispectral imagery (R and NIR bands) into QGIS.
- Load the field boundary layer if available to limit the analysis to the area of interest.
2. Calculate NDVI
- Use the Raster Calculator to compute the NDVI using the formula: (NIR - Red) / (NIR + Red)
- Save the output as a new raster layer (e.g. "NDVI").
3. Classify NDVI into Zones
- Use the Reclassify by Table tool to classify NDVI values into three or more categories (e.g., low, medium, high vegetation health).
- Define thresholds based on the NDVI range (e.g. low: 0-0.3, medium: 0.3-0.6, high: 0.6-1.0).
- Save the output as a new raster layer (e.g. "NDVI_Zones").
4. Create a Prescription Map
- Convert the classified NDVI raster into vector polygons using the Raster to Polygon tool.
5. Export Results
- Export the prescription map as a shapefile or GeoJSON for use in variable rate application equipment.